Sales forecasting is the process of estimating future sales revenue based on historical data, market trends, and other relevant factors. Accurate sales forecasting is essential for businesses to allocate resources effectively, set realistic goals, and make strategic decisions.
This article will explore six proven sales forecasting methods that can help you predict revenue with greater accuracy. We’ll also discuss how modern tools can enhance your forecasting efforts and provide best practices for implementation.
The Fundamentals of Sales Forecasting
Before diving into specific methods, let’s establish a clear understanding of sales forecasting and its importance in business planning.
Sales forecasting is the process of predicting future sales performance based on various data points and analysis techniques. It involves estimating the amount of revenue a company expects to generate within a specific timeframe, typically a month, quarter, or year.
Accurate sales forecasting offers several key benefits:
- Improved resource allocation
- Better inventory management
- More effective budgeting and financial planning
- Informed decision-making for growth strategies
- Enhanced investor and stakeholder confidence
Despite its importance, sales forecasting comes with its share of challenges:
- Data quality and consistency issues
- Market volatility and unexpected events
- Changes in customer behavior and preferences
- Internal factors such as sales team turnover or product changes
To overcome these challenges and improve your forecasting accuracy, it’s essential to use the right tools and methods. One way to enhance your forecasting process is by connecting your CRM data directly to your spreadsheets. Learn more about how to connect Salesforce to Google Sheets for seamless data integration.
6 Effective Sales Forecasting Methods
Let’s explore six proven sales forecasting methods that can help you predict revenue with greater accuracy.
1. Opportunity Stage Forecasting: Mapping Your Sales Pipeline
Opportunity stage forecasting is a method that assigns probability percentages to each stage of your sales pipeline. This approach helps you estimate potential revenue based on where deals are in the sales process.
How to implement:
- Define your sales pipeline stages clearly
- Assign probability percentages to each stage
- Calculate potential revenue for each opportunity
- Sum up the weighted values for your forecast
Best practices:
- Regularly review and update stage probabilities
- Use historical data to refine your percentages
- Consider deal size and complexity when assigning probabilities
Pros:
- Provides a structured approach to forecasting
- Easy to implement with most CRM systems
- Offers visibility into pipeline health
Cons:
- Can be overly optimistic if not calibrated properly
- May not account for external factors affecting deal progression
Real-world example: A software company uses opportunity stage forecasting to predict quarterly revenue. They assign the following probabilities: Qualified Lead (10%), Demo Scheduled (30%), Proposal Sent (60%), Contract Negotiation (80%), Closed Won (100%). By summing the weighted values of all opportunities, they can estimate their likely revenue for the quarter.
2. Length of Sales Cycle Forecasting: Timing is Everything
This method focuses on the average time it takes to close a deal, using historical data to predict when future deals are likely to close.
Implementation steps:
- Calculate the average sales cycle length for your products or services
- Track the start date of each opportunity in your pipeline
- Estimate close dates based on the average sales cycle
- Project revenue based on these estimated close dates
Best practices:
- Segment your data by product type, deal size, or customer segment for more accurate predictions
- Regularly update your average sales cycle length
- Consider factors that might accelerate or delay the sales process
Pros:
- Provides a time-based perspective on revenue
- Helps identify bottlenecks in the sales process
- Useful for resource planning and cash flow projections
Cons:
- May not account for variations in individual deals
- Can be less accurate for new products or markets
Real-world example: A manufacturing company sells industrial equipment with an average sales cycle of 90 days. They use this information to forecast which deals in their pipeline are likely to close within the next quarter, allowing them to set realistic targets and allocate resources accordingly.
3. Intuitive Forecasting: Leveraging Sales Team Expertise
Intuitive forecasting, also known as qualitative forecasting, relies on the experience and judgment of your sales team to predict future sales.
Implementation process:
- Gather input from individual sales representatives
- Hold team meetings to discuss and refine predictions
- Consider factors like market trends, competitive landscape, and economic conditions
- Combine individual forecasts into an overall prediction
Best practices:
- Provide a structured format for collecting forecasts
- Encourage honest and realistic assessments
- Combine intuitive forecasts with data-driven methods for balance
Pros:
- Incorporates valuable on-the-ground insights
- Can account for factors not captured in historical data
- Builds engagement and accountability within the sales team
Cons:
- Subject to individual biases and optimism
- May lack consistency across different team members or periods
Real-world example: A real estate agency uses intuitive forecasting to predict monthly sales. Each agent provides their estimate based on current listings, market conditions, and personal pipeline. The sales manager then combines these inputs with historical data to create a final forecast.
4. Historical Forecasting: Learning from the Past
Historical forecasting uses past sales data to predict future performance, assuming that past trends will continue.
Implementation steps:
- Collect and organize historical sales data
- Identify patterns and trends in the data
- Apply statistical methods (e.g., moving averages, trend analysis)
- Project these patterns into the future
Best practices:
- Use a sufficient amount of historical data for accuracy
- Account for seasonality and cyclical patterns
- Regularly update your model with new data
Pros:
- Based on actual performance data
- Relatively simple to implement
- Effective for stable markets and established products
Cons:
- May not account for market changes or new initiatives
- Less effective for new products or rapidly changing markets
Real-world example: An e-commerce company uses historical forecasting to predict holiday season sales. By analyzing data from previous years, they identify growth rates, seasonal peaks, and the impact of promotional events. This information helps them forecast inventory needs and staffing requirements for the upcoming holiday season.
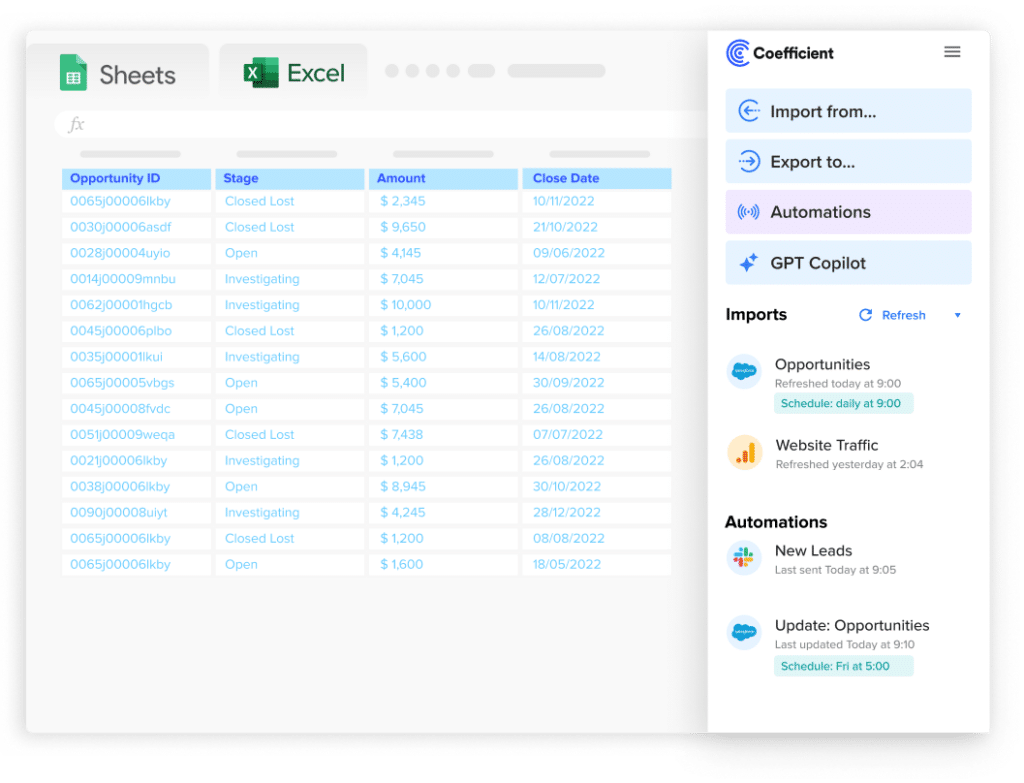
Stop exporting data manually. Sync data from your business systems into Google Sheets or Excel with Coefficient and set it on a refresh schedule.
Get Started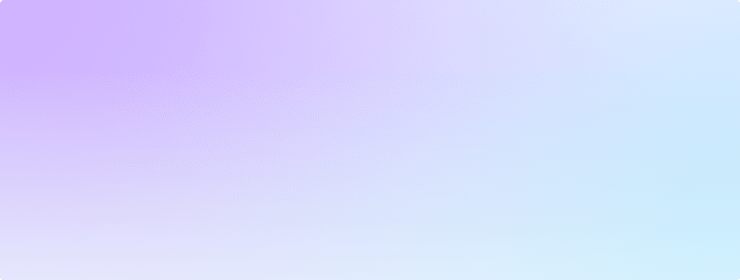
5. Multivariable Analysis Forecasting: A Holistic Approach
Multivariable analysis forecasting considers multiple factors that can influence sales, creating a more comprehensive prediction model.
Implementation process:
- Identify key variables that impact sales (e.g., economic indicators, marketing spend, competitor actions)
- Collect data on these variables
- Use statistical techniques to determine the relationship between variables and sales
- Create a model that incorporates these relationships to forecast future sales
Best practices:
- Regularly review and update the variables in your model
- Use statistical software or advanced spreadsheet functions for complex analyses
- Validate your model with historical data before using it for forecasting
Pros:
- Provides a more nuanced and potentially accurate forecast
- Can identify key drivers of sales performance
- Adaptable to changing market conditions
Cons:
- Requires more data and analytical expertise
- Can be complex to implement and maintain
- May be overkill for smaller businesses or simple sales processes
Real-world example: A global consumer goods company uses multivariable analysis to forecast sales across different regions. Their model incorporates factors such as GDP growth, consumer confidence index, marketing expenditure, and competitor pricing. This comprehensive approach allows them to adjust their strategies based on regional economic conditions and market dynamics.
6. Test-Market Analysis Forecasting: Learning from Small-Scale Trials
Test-market analysis involves conducting small-scale trials or launches to gather data for forecasting larger-scale sales performance.
Implementation steps:
- Select a representative test market or segment
- Launch your product or campaign in the test market
- Collect and analyze sales data from the test
- Extrapolate results to forecast performance in larger markets
Best practices:
- Choose test markets that closely resemble your target market
- Control for external factors that might skew results
- Allow sufficient time for the test to yield meaningful data
Pros:
- Provides real-world data for new products or markets
- Can uncover unexpected challenges or opportunities
- Reduces risk of full-scale launches
Cons:
- Can be time-consuming and costly
- Results may not always scale accurately to larger markets
- Competitors may become aware of your plans
Real-world example: A fast-food chain wants to introduce a new menu item nationwide. They first launch it in three test markets with different demographics. By analyzing sales data, customer feedback, and operational challenges in these markets, they can more accurately forecast the potential success and required resources for a national rollout.
Enhancing Your Sales Forecasting with Coefficient
While these forecasting methods are powerful on their own, combining them with advanced tools can significantly improve your accuracy and efficiency. Coefficient is a spreadsheet add-on that can transform your sales forecasting process by:
- Syncing real-time data from your CRM and other business systems directly into your spreadsheets
- Automating data updates, ensuring your forecasts are always based on the latest information
- Enabling easy collaboration and sharing of forecasts across your team
- Providing powerful analytics capabilities within familiar spreadsheet environments
Coefficient’s Excel integration and Google Sheets integration allow you to leverage the power of these popular spreadsheet tools while enhancing them with advanced data capabilities.
Ready to take your sales forecasting to the next level? Get started with Coefficient today and experience the power of real-time, automated data integration for more accurate and efficient forecasting.
Further Reading
- How to Connect Your CRM to Google Sheets
- Excel Integration with Coefficient
- Google Sheets Integration with Coefficient
Frequently Asked Questions
How to do a 12-month sales forecast?
To create a 12-month sales forecast, start by selecting an appropriate forecasting method based on your available data and business needs. Use historical data as a baseline, and adjust for known factors like seasonality, planned promotions, or market trends. Coefficient can help by automating data imports from your CRM and other systems, ensuring your forecast is based on the most up-to-date information.
How to create a 12-month forecast?
Creating a 12-month forecast involves gathering historical data, identifying trends and patterns, and projecting these into the future while accounting for known variables. Coefficient’s automation features can streamline this process by keeping your data current and enabling easy updates to your forecast as new information becomes available.
What is the 3-9 forecast template?
The 3-9 forecast template is a sales forecasting tool that provides visibility for the next 3 months (near-term) and 9 months (long-term) of projected sales. It typically includes detailed forecasts for the next quarter and broader projections for the following three quarters. Coefficient’s template capabilities can help you create and maintain a 3-9 forecast with real-time data updates and collaborative features.
How to create a sales forecast spreadsheet?
To create a sales forecast spreadsheet, start by outlining your forecasting method and required data points. Set up columns for historical data, projections, and any relevant variables. Coefficient’s spreadsheet integration capabilities allow you to pull live data from your CRM and other systems directly into your forecast, ensuring accuracy and saving time on manual updates.